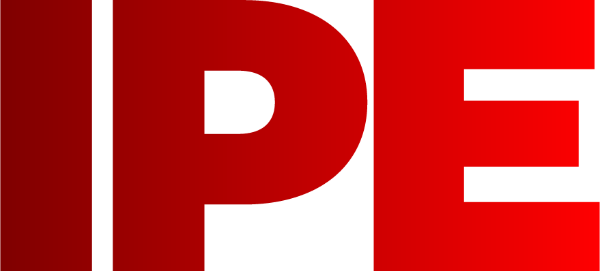
![]() |
Edward Lowton
Editor |
![]() ![]() |
Predictive maintenance pushes technology boundaries
25 October 2018
Few things can damage the financial stability of a manufacturing facility more than unexpected downtime. On average, manufacturers suffer with 30% or more downtime during their scheduled production time. However, there are ways to reduce the risk.
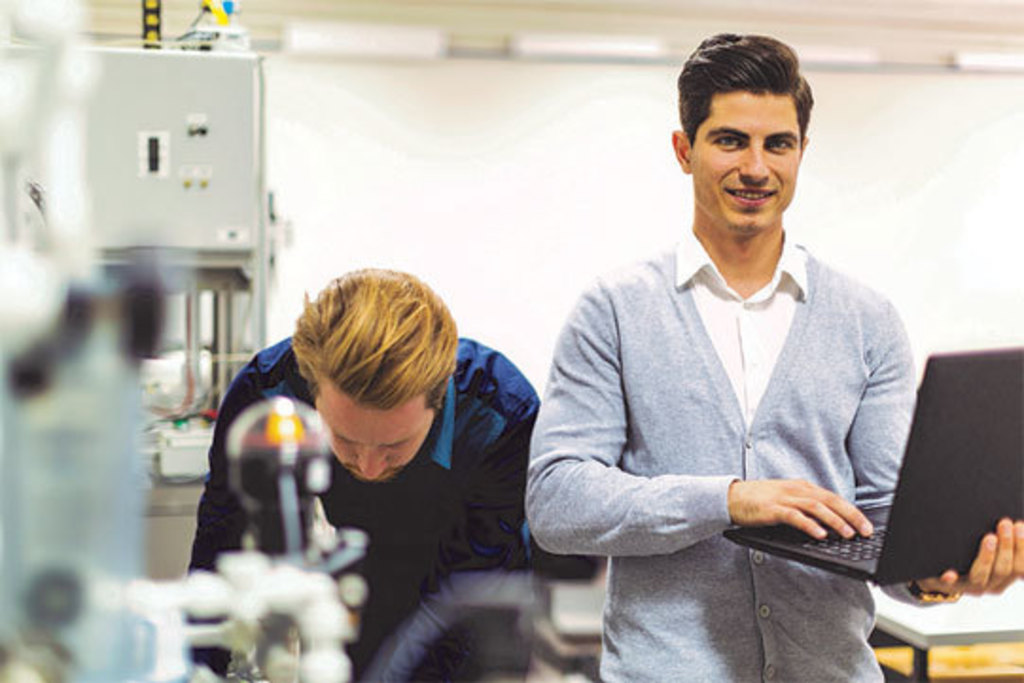
The automotive industry has long been at the forefront of applying new automation technologies, but the industry is also at the forefront of industrial network modernization. Downtime can cost up to £17,000 per minute.
Traditionally, industrial maintenance has been done on a fixed schedule, replacing items at constant intervals. Constant intervals do not take into consideration the unique circumstances of a machine being maintained, so although general purpose schedules are convenient from the viewpoint of ordering spares, they risk wasting money by replacing parts that are still operational.
Predictive maintenance, on the other hand, aims to get the most life out of equipment while minimising the risk of failure. Increasingly, it involves gathering large quantities of data. While some of this will be existing data, such as maintenance or warranty records, adding data sources such as sensors on the equipment will also be important to build the big picture.
One of the biggest challenges is gathering and interpreting unstructured data, such as free text in maintenance records, design specs, test data from failed equipment, or even comments on social media or Google searches. The most intelligent data analytical automation software will also provide graphical visualisation of production data and generate customised statistical reports.
Having collected all the data, the next step involves using data analytics to make sense of the data, and then figuring out predictive rules that will become the basis for a predictive maintenance model. Once the model is built, it must be tested and progressively refined. But a bad model is better than no model, albeit only marginally – and with progressive refinement via a continuous learning loop the model can be updated based on on-going results. For example, if a component fails after being used for a specific product run, pattern recognition can identify the stresses that are unique to that run that could have caused the failure.
Inside AWNC, the Toyota transmission plant in North Carolina, a recent upgrade includes a new MES, inventory management, predictive maintenance and quality systems that transmit all data for collection and analysis over a secure, Cisco-enabled Wi-Fi network.
Video data
According to test and measurement company HBM, video use is accelerating in data collection. Video cameras are already used in many test and measurement applications in addition to data collection with traditional tactile sensors.
“There is no longer any question that recording video data in parallel to tactile sensors or digital bus signals is becoming more and more attractive to users,” says Christof Salcher, Product Manager Instrumentation at HBM. “Video supports traditional sensor data and is becoming a valuable source of additional information.
Caption
Video use in data collection is on the increase according to a recent study by HBM.
Zenon from Copa-Data is software for industrial automation in which machines are controlled, monitored and optimized using open communications.
- Smart monitoring for maintenance and part quality
- Digitising legacy systems: Challenges & opportunities
- Slow Speed Bearing Inspection with Ultrasound
- Fire Safety Matters wins major media award
- InVMA to provide ESAB® Welding & Cutting Products with IoT services and consultancy
- First judges announced for IP&E Awards
- Technical experts in maintenance and reliability announced as key speakers in new-look Maintec seminar programme
- Klüber Lubrication receive prestigious innovation award
- Providing practical solutions to everyday maintenance challenges
- Nineteen Group acquires Western Business Exhibitions
- Three minutes with Bosch Rexroth
- Smart monitoring for maintenance and part quality
- Modern Maintenance Matters
- The patchy case of maintenance in the Food & Drink industry
- Five essential health & safety updates for business leaders in 2018
- Adapting the supply chain to maintenance needs
- Bosch Rexroth confirms event partnership for Maintec 2018
- Leading trade body BSIF announces support for Maintec 2018
- PTC and InVMA supporting a winning project at Birmingham City University Innovation Fest
- InVMA enables delivery of Connected Healthcare Devices.