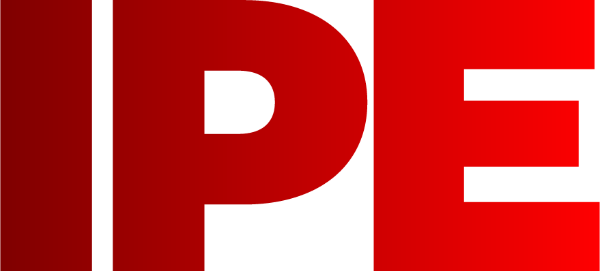
![]() |
Edward Lowton
Editor |
![]() ![]() |
Home> | Efficient Maintenance | >Condition monitoring | >Overcoming predictive maintenance scepticism |
Overcoming predictive maintenance scepticism
10 March 2021
Despite the numerous and easily quantifiable advantages that can be gained, when it comes to implementing predictive maintenance, there are still some who have their doubts. Philipp Wallner looks at what lies behind the scepticism and how to overcome it
PREDICTIVE MAINTENANCE enables engineers to anticipate and respond to potential equipment malfunction before it becomes a problem. It is an invaluable set of tools to proactively schedule repairs and minimise overall disruption to factory floor operations which ultimately benefit the bottom line.
Yet for all the advantages predictive maintenance offers, doubts about whether this technology delivers measurable benefits still exist in the industrial plant and equipment industry.
So, what is behind this scepticism? Some are sceptical because they have difficulty determining return on investment and are unclear about whether they have the right data, or enough equipment failure data, to achieve a working algorithm.
Engineers may too have an inaccurate understanding of predictive maintenance. The term can sometimes be misunderstood as being a ‘black box’ solution, whereby operational data from machines is fed into an application, and an algorithm provides the remaining useful life of the equipment. This representation disregards the role of domain knowledge in developing algorithms to detect and predict downtime.
To achieve more accurate predictive maintenance algorithms, organisations should deploy individuals with expertise in both engineering and data science. Engineers should work with data scientists to generate the necessary failure data from equipment to improve how algorithms are trained.
The use of simulation software tools can ease this vital process. The software helps employees who are not as experienced in implementing predictive maintenance with a variety of easy to apply techniques for collecting data and then training algorithms.
Simulation can help ensure predictive maintenance algorithms are properly trained and powerful, even when less real-world data can be fed into them. This is important because to fully train algorithms, engineers and data scientists need adequate failure data. However, data about malfunctioning machinery is not always available. It is expensive and inefficient to intentionally run equipment to fail for the purposes of collecting such insights which is why simulation software models can play a crucial role by simulating how industrial assets function in various test scenarios. This enables teams to produce failure data that can then be used to train algorithms, avoiding the need to find or generate failure data in real-world settings.
Successful predictive maintenance use cases
There are many companies that have successfully benefited from this approach to customise predictive maintenance software to their specific needs. This includes energy technology company Baker Hughes and packaging and paper goods manufacturer Mondi.
Baker Hughes developed pump health monitoring software leveraging data analytics for predictive maintenance. In doing so, the oil field service company reduced costs related to equipment downtime by 40%. It also cut down the need for additional onsite trucks.
Mondi used software tools to create an equipment health monitoring and predictive maintenance application to identify possible machinery issues before they occur. It was able to get its system ready within six months.
These examples show how companies can overcome challenges of predictive maintenance, from not being able to train accurate algorithms to not having enough failure data. Software simulation tools simplify the process by making predictive maintenance algorithms more powerful and ensuring less real-world data is needed to train them. Software also enables individuals with less experience in data science to carry out different techniques to preprocess data and train predictive models based on the data available.
The current state of predictive maintenance
Today, most companies have predictive maintenance algorithms onsite and close to equipment, such as an edge server that amasses data locally in a production facility or from wind turbines. Over the next five years, organisations should expect to see a new era of software functionality on production systems. This will stem from the continually increasing calculation power of industrial controllers and edge computing devices as well as advancements in cloud computing. Predictive maintenance will evolve to take into consideration data that is not just from single machines but from assets across multiple factory locations and from different vendors. In addition, AI-based algorithms will deploy on non-real-time systems as well as real-time platforms such as programmable logic controllers, depending on the requirements.
The most impactful use of predictive maintenance will be feeding data into a single cloud platform from equipment from sources around the world. Cloud computing allows manufacturers to collect data from numerous areas, not just from local sites, and efficiently train predictive maintenance algorithms. There will continue to be some scepticism around data security and ownership, but cloud-based predictive maintenance will become reality.
Predictive maintenance has undeniable advantages for companies applying this set of tools to their production lines. Organisations that have yet to determine how predictive maintenance fits into their business models and can be monetised are at a disadvantage to their competitors. With resources available to enable the pairing of domain expertise and machine learning, and simulation tools to generate failure data and train more powerful algorithms, predictive maintenance and its benefits are within reach.
Philipp Wallner is industry manager, industrial automation and machinery at MathWorks