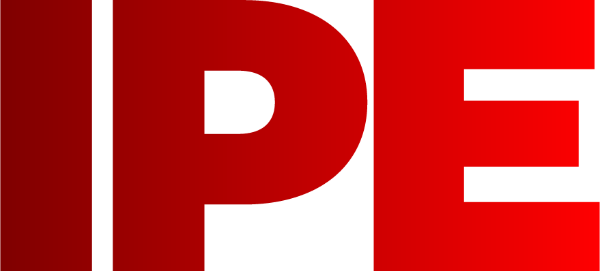
![]() |
Edward Lowton
Editor |
![]() ![]() |
Home> | Energy Management | >Sustainable energy | >Can machine learning and AI transform energy efficiency? |
Can machine learning and AI transform energy efficiency?
29 September 2023
Many are surprised to learn that AI and machine learning are already making a significant difference to the way businesses are able to improve energy efficiency, helping identify energy wastage which may be hiding in plain sight, says David Sing

THERE'S PROBABLY never been a more important time for industry to get ‘energy fit’ as part of its wider sustainability goals. As manufacturers come to terms with scaled-back energy support for most non-domestic users, there’s growing interest in how technology could help businesses bear down on consumption and cost. And when we say technology, we mean machine learning and artificial intelligence (AI) in particular.
AI is attracting a huge amount of attention at the moment, both good and bad, but the question we are increasingly being asked at Energy Assets is whether AI and machine learning can make a difference to energy efficiency.
Many are surprised to learn that it’s already here, and making a significant difference to the way businesses are able to improve efficiency and, in particular, drive out energy waste that can often be hiding in plain sight.
That said, to be truly effective, AI-informed machine learning can only be as good as the data that’s fed into it. So before, companies look to AI to improve their energy profile, there are some basic steps to be taken to lay the foundations for machine learning, including making sure that consumption data is being captured in granular detail via existing automated meter reading systems.
Of course, some businesses will already employ advanced AM&T portals, such as our own WebAnalyser, to monitor and report on consumption, run bespoke reports, and set automated alerts for unusual spikes in consumption.
This may be more than adequate for many users, but the advance of AI and machine learning does provide an opportunity to go further, both to bear down on cost and support carbon reduction strategies.
Heavy data lifting
At Energy Assets, we recognised the potential impact of AI and machine learning a few years ago and launched AMR DNA, powered by kWIQly, on the back of the availability of hour-hourly metered data.
What’s really impressive about machine learning is its ability to progressively learn what optimal performance looks like in a building across all its consumption profiles and to produce a checklist of actions that will improve energy efficiency. And unlike us mere mortals, it never stops.
Day in, and day out, this tool is automatically assimilating meter data and interpreting it in the context of building function and external factors (weather, occupancy levels). This creates ‘fingerprints’ of consumption - and because the system is AI-led, it learns to ignore outcomes that are irrelevant, mistaken or due to bad data.
Crunching data on this scale manually, particularly across multi-building portfolios, would require an army of analysts - but with machine learning, this can be achieved in a quick time.
Often, it’s a question of spotting improvement opportunities hiding in plain sight, such as equipment running needlessly or heating controls incorrectly set - and machine learning is the perfect tool to do that.
Moreover, the value of metered data will only increase with the Market-wide Half-Hourly settlement (MWHH) reform due by 2025, which will enhance opportunities for I&C users to negotiate demand side response incentives and preferential time-of-use tariffs from their energy suppliers.
The science of energy waste
Pinning down portfolio-wide energy waste and improvement opportunities requires rock-solid benchmarks to compare like-with-like. Firstly though, it’s worth focusing attention on what we mean by ‘waste’ because it comes in many forms:
- Precedent waste - where a building does not perform as well as it has in the past (and noting that operational contexts and use-cases of a building will change and must be re-learned).
- Routine waste - where a building can be shown to systematically use energy that cannot be necessary or comfortable (e.g., if heating is maximised at +5°C, since colder weather requires more heating; a combination of discomfort or waste exists at all temperatures between -5°C and +5°C).
- Peer or benchmarked waste - where a building does not comply with its peers (for example, sets of comparable buildings are expected to have similar balance temperatures, night-setback loads and apparent occupancy patterns).
The analytics engine inherent in AMR DNA has several report modes to save energy managers’ time. These include establishing achievable targets by KPI and weather condition for each meter, forecasting future use and identifying where changes have taken place; and diagnosing priority and cause of savings opportunity.
However, it is also important to acknowledge (and quantify) the impact of efforts made. Accordingly, as illustrated in this article - proof-of-savings reports can include graphic comparisons of prior performance and any change at the click of a button.
Energy managers will, of course, use their professional expertise and knowledge of each building to apply machine learning data reports to those areas where action can positively impact on efficiency.
Often, it’s a question of spotting improvement opportunities hiding in plain sight, but sometimes these can be the hardest to identify because they are ingrained in a building’s legacy performance and can be easily overlooked.
Fortunately, machine learning can help unpick waste by thorough historical analysis of half-hourly meter data and interpreting it in the context of how and when the build is operational, taking into account external factors such as weather and occupancy levels.
When primed with this information, these tools can assist managers in optimising energy performance across their entire building portfolio.
BOXOFF
Energy consumption comparison report
This report compares a period of 796 days from 2020-01-17 to 2022-03-22 with a period of 298 days after the implementation of machine learning changes from 2022-03-23 to 2023-01-14. Each period has models of consumption in response to outside air temperature for each day of the week.
These are then applied to a typical annual weather pattern for the location to come up with expectations of annual performance on the new and old basis. The difference represents a saving which might be expected if the performances prior to and after changes were replicated for the hypothetical typical year:
Before kWh = 1,522,658; After kWh = 1,044,713; Saving Energy 477,945 kWh; Saving Percentage 31.4 %.
The bottom line represents the cumulative deviation from expected consumption, where ‘Up’ is wasteful and ‘Down’ is ahead of expected. The turquoise section represents the period benefiting from machine learning.
David Sing is MD, metering and data at Energy Assets
For more information:
Tel: 0333 9000 405