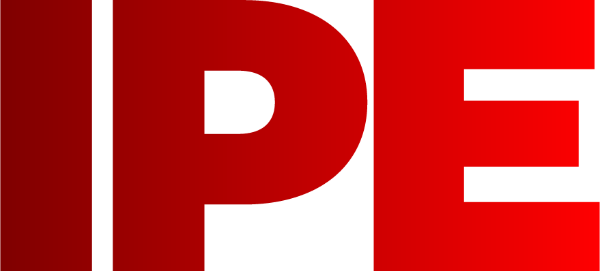
![]() |
Edward Lowton
Editor |
![]() ![]() |
Home> | Efficient Maintenance | >Industry 4.0 | >How machine learning is transforming predictive maintenance |
How machine learning is transforming predictive maintenance
31 January 2019
IP&E consultant editor, Andy Pye, takes a look at some of the findings of Banner Engineering's latest white paper.
Predictive maintenance is becoming essential to the smart factory. The ability to accurately track machine performance and anticipate failures before they occur is helping manufacturers improve overall equipment effectiveness and reduce wasted time and costs.
Traditionally, plant managers relied on preventative maintenance schedules provided by a machine’s manufacturer, including regularly replacing machine components based on a suggested timeline. However, these timelines are only estimates of when the machine will require service, and the actual use of the machine can greatly affect the reliability of these estimates.
A leading solution for predictive maintenance is condition monitoring which plays a key role in predictive maintenance by allowing users to identify critical changes in machine performance. One important condition to monitor is vibration. Machine vibration is often caused by imbalanced, misaligned, loose, or worn parts. As vibration increases, so can damage to the machine. By monitoring motors, pumps, compressors, fans, blowers, and gearboxes for increases in vibration, problems can be detected before they become severe and result in unplanned downtime.
Vibration sensors typically measure RMS velocity, which provides the most uniform measurement of vibration over a wide range of machine frequencies and is indicative of overall machine health. Another key data point is temperature change (overheating). Machine learning takes this information and automatically defines a machine’s baseline.
However, collecting machine performance metrics is only the beginning. The ability to accurately interpret and communicate this data is essential for system reliability, and that’s where machine learning comes into play. Condition monitoring with machine learning will recognise the machine’s unique baseline of vibration and temperature levels and automatically set warning and alert thresholds at the appropriate points. This makes the condition monitoring system more reliable and less dependent on error-prone manual calculations.
Wireless condition monitoring is easy to deploy quickly. The ability to monitor machines in inconvenient locations allows for more comprehensive monitoring and increased reliability.
Based on a white paper from Banner Engineering: "Predictive Maintenance Trends: How Machine Learning is Transforming Machine Maintenance”
Image
Predictive maintenance is becoming essential to the smart factory. The ability to accurately track machine performance and anticipate failures before they occur is helping manufacturers improve overall equipment effectiveness and reduce wasted time and costs.
- Gardner Denver to combine with Ingersoll Rand's industrial segment
- 'Don't delay apprentice recruitment plans', urges Make UK
- Nuclear fusion facility under construction
- Rethinking access security for workplace vehicles
- Manufacturing outlook worsens amidst Brexit despair
- Green jobs need to be more than a figment of politicians' imagination, says GMB
- World demand for food processing machinery expected to rise to $73bn in 2019
- Optimising machine reliability
- The Big Bang Competition calls for inspiring young innovators
- Call for papers for CM 2020 conference
- Technology and Open Standard data enabling smart maintenance
- Broadband sensors: Pinpointing leaks in compressed air systems
- Mechanised welding, cutting and Industry 4.0
- Tomorrow’s tech is here today
- Investment in infrastructure, innovation and people
- Getting to 4.0 – one hard-won gain at a time
- Digital transformation in the chemical industry: White paper
- Digital industry around the world
- Guidance via AR
- Real-time data helps reduce costs and machine downtime