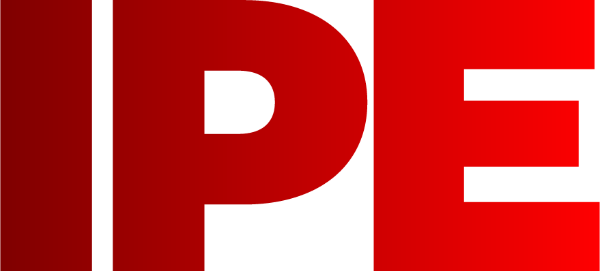
![]() |
Edward Lowton
Editor |
![]() ![]() |
Leveraging data for predictive maintenance
23 September 2021
Data can elevate the maintenance function from being a quick fix to keep production lines running, to being a vital tool for enterprise success. Dan Rossek explains how to raise your maintenance strategy to this level by accessing and repurposing data that already exists.
UNPLANNED DOWNTIME is the enemy of production, and because most manufacturing systems work as part of a wider ecosystem, even the failure of a single component can impact on productivity across the enterprise – affecting upstream and downstream operations. It can also have an adverse effect on product quality or could result in costly product losses. Ultimately, it can lead to lost revenue and damage to brand reputation at a business level.
The most common cause of machine fatigue is mechanical component failure; for example, bearing wear, air cylinder failure, misalignment of guides and machine jams. Too many manufacturers still rely on the most basic maintenance strategy – run-to-failure – and experience unexpected downtime events as a result.
A better solution is preventative maintenance, where components are swapped out at set times in line with their expected operational lifetime. While this reduces unplanned stoppages, the downside is that the component may still have more life left in it, meaning that a preventative strategy does not always align well with an organisation’s sustainability goals. Replacing components before they reach the end of their useful lives can also be a costly solution.
The human cost As many industries start the journey towards smarter factories, it is also important to keep in mind the human impact of maintenance. Digital solutions can offer many benefits for maintenance teams, helping to relieve the huge pressure placed on them when there is a stoppage. The time taken to get a system back online will dictate how costly the downtime is to the enterprise. This can lead to time being prioritised over the quality of a fix, resulting in frequent machine stoppages.
While it must be accepted that no maintenance strategy can guarantee 100% machine uptime, both preventative and predictive solutions can help reduce the physical pressures on the team, as fixes can be planned in advance and undertaken during scheduled downtimes.
Starting the predictive journey
In today’s digital world, predictive maintenance can be achieved more easily than you may think. Essentially, a predictive maintenance strategy can utilise already-available data from components and analyse this information, highlighting spurious signals which can indicate a potential failure. Take, for example, vibration or temperature. As most components will run at a baseline vibration level or a regular operating temperature, monitoring these elements makes it possible to identify changes, which might be due to a component starting to fail. Looking more closely at the vector or velocity of change makes it possible to predict a timeframe to failure.
The first step on a predictive maintenance journey is to identify critical elements of the process. What historically are the major causes of unplanned stoppage and where on the system do they occur? This will enable you to create a baseline of the data collection capabilities of your systems.
Often, the critical elements will be motors and mechanical elements such as air solenoids. Adding predictive maintenance solutions onto these components is usually an easy task, and often there is no requirement for networking to wider systems.
Furthermore, there is usually no need to add more technology, just data recording capability. Many inverter drives, for example, will already include current monitoring functionality, so the data is available. If your inverter doesn’t have this functionality, a simple solution is to deploy current transformers onto the motors. Vibration sensors can identify increased or changing levels of vibration which can help predict a failure, while air solenoids contain position sensors which can be used to determine the condition of the device by analysing the time it takes to retract and extend.
One challenge, however, is understanding that there is a need to interpret the data differently. For example, most machines will record the stop and start positions of the solenoid, but for predictive maintenance, it is necessary to record the time it takes to go from one point to another. This time should be consistent and any variance may indicate an issue with the cylinder.
With your data gathering solutions identified, the next step is to assess data collection options. Some ageing control systems will have no data capture capability, but there will be little appetite to upgrade to a more modern architecture just to handle this task. A good alternative is to add a secondary layer of automation architecture to collect data and interface to the supervisory system. This is not a costly undertaking, as there is no requirement for any control functions.
Finding your level
There are various levels of predictive maintenance, and once you start receiving huge amounts of data, the levels of complexity will increase. It can also be difficult to filter out readings that relate to normal interruptions that can cause data fluctuation – periodic loading of a machine, for example, can cause a spike in vibration, or there may be a repetitive process action which causes increases in vibration and thus a variation in the measurements.
Artificial intelligence (AI) technology can offer a good solution here; it can quickly learn the measurement patterns and filter out regular activities while highlighting any unexpected step changes. Edge-based AI solutions are great at monitoring information from a relatively small number of datasets in real time to provide instant data analysis that allows equipment to be regularly adjusted, helping ensure it is always working at optimum capacity. Alternatively, a cloud-based AI solution escalates information from a greater number of datasets to the cloud, allowing large amounts of data to be analysed over longer periods. No one AI solution is better than another; it simply depends on the needs of the particular application.
The growing quantity of data on the plant floor and the expanding availability of simple analysis tools have made the maintenance function increasingly important in helping to raise productivity. Automation vendors who understand the vital role that predictive maintenance can play are already developing enabling solutions which integrate maintenance functionality into their offerings.
The latest generation of Omron robots, for example, includes integrated maintenance functionality to make data easily accessible to simplify predictive maintenance. In the future, users should expect maintenance to become an integral function of many more components.
Sensor technology is also adapting to meet the need for increased maintenance functionality. While vibration sensors already have an analogue output, it is at a frequency that is far too high for most controllers to record. Today, sensors are being developed which incorporate an interface to enable the collation and bulk sending of data to analytics packages.
In the not-too-distant future, rather than having to deploy or analyse sensor technology just for maintenance purposes, this function will be integrated into existing systems. This will help to transform the maintenance function to a position where it can provide a positive contribution to enterprise success by increasing productivity and minimising waste.
Dan Rossek is regional marketing manager at Omron
- AC servo system with motion safety functionality
- Ensuring continuity of service in water processing
- Keeping safety simple
- Speedy vision system
- Cobot welding cell
- Agile cobot redeployment enabled
- New FH vision system with AI defect detection
- OMRON introduces new MD-series autonomous mobile robots
- Making FMCG manufacturing more sustainable through intelligent energy management
- Industry 4.0: Implications for sensor technology
- No related articles listed