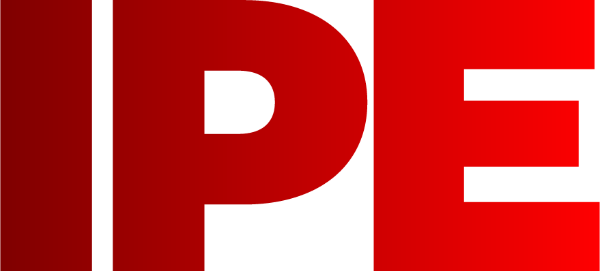
![]() |
Edward Lowton
Editor |
![]() ![]() |
Home> | Plant, Process & Control | >Industry 4.0 | >Did we celebrate autonomous quality inspection too soon? |
Did we celebrate autonomous quality inspection too soon?
03 May 2023
While there have been a number of optimistic predictions about the potential for fully autonomous quality inspection, several challenges remain to be addressed, explains Zohar Kantor

IN MANY industries, advocates of artificial intelligence and autonomous technology are quick to promise sweeping transformation and fully autonomous solutions. However, the optimists usually promise more than they can deliver and soon find the engineering challenges are greater than they first realised.
In 2013, Elon Musk said, "it's a bridge too far to go to fully autonomous cars". Although the world of driverless vehicles has moved on significantly since this admission, it was a belated recognition that the Tesla CEO had under-estimated the challenges of operating a vehicle without a human being in the driver’s seat.
Having spent a few years working in the field of machine vision, I can see many parallels. With the sudden influx of claims about artificial intelligence and autonomous solutions, quality managers were left with bold promises of fully autonomous quality inspection - the expectation of a system that can operate flawlessly without an operator guiding its setup at every step of the installation.
The complexity minefield
The challenge of introducing autonomous machine vision is best examined through the lens of a given use-case. Let’s return to the driving analogy. You can easily have a vehicle that drives, without a human driver in control, if the challenge is to slowly drive along a straight line in a closed environment. Autonomous construction vehicles that move material from one area of a quarry to another come to mind.
If, however, you are trying to develop a passenger vehicle that can navigate a complex urban environment, the task is completely different. The same applies with machine vision technology. Whether or not we can easily automate something depends largely on the use case and the level of complexity this involves.
For some applications, where the complexity of capturing an image is low, a straightforward smart sensor and a simple camera with simple white lighting is enough. In other instances, where a special elimination profile other than white light or HDR camera is required, we might classify the use case as high on the scale of complexity of image acquisition.
Then there is the second major challenge, the complexity of defect inspection. Some defects are much harder to spot or categorise than others. For a simple use case, where the complexity of defect inspection can be classified as low, a defect might be detected by, for example, detecting presence or component polarity. In contrast, detecting a minor scratch on a metal surface after grinding is not an easy task and can be classified as highly complex in comparison. Another example of use cases where complexity of defect inspection is high might be where there is a need to detect each unique defect type (class) and applying special criteria, such as size or tolerance.
How many images do you need?
Autonomous quality inspection solutions that claim to be able to point-and-shoot with a camera and integrated software with just a small sample of around 50 good images are very ambitious. However, it might be achievable in use cases where complexity of acquisition and complexity of defect inspection is low. In a simple acquisition set up with consistent product texture and deterministic defects, this might be realistic.
However, for many businesses it is a different story. In cases where there are many surface nuances, highly complex defects with specific tolerances, in addition to “permissible defects” which are accepted, the only way to train a model is by tagging huge amounts of data sets. Expecting an Autonomous Machine Vision to self-learn more sophisticated use cases is simply not going to work. Much like driverless cars in 2013, it’s a bridge too far.
Although there is no escaping the need for having lots of images for complex use cases, greater automation still holds the key to improve quality inspection. A hybrid approach is to use sophisticated AI models to automate the learning process and to allow the user to provide feedback when needed to guide the learning process in the right direction. The challenge is to minimise the amount of guidance, but not to eliminate it.
The premise is that the user holds a high level of knowledge pertaining to the product target, using it to guide the learning process is therefore a win-win; automating the process while allowing the user to provide guidance where needed. That’s why at LeanAI we are focused on building a solution that significantly reduces the effort required to train and retain inspection systems.
Zohar Kantor is vice president of sales at Lean.AI
For more information:
Tel: +972 54-655-4332
- KC Movers on the move with eCapital
- Entry-level plant trailer
- Making the case for GRP
- Irish manufacturer wins Investment of the Year award
- Workplace safety at risk due to de-regulation of health & safety policies
- Bridging the gap between academia and industry
- MAINTAINING MOMENTUM
- Nominations open for 2021 Queen Elizabeth Prize for Engineering
- Applications for Personal Guarantee Backed Finance rose 82% in 2024 as SME manufacturers sought to sustain their businesses
- thuba extends explosion-proof signalling device range with buzzer
- Introducing Industry 4.0 to SMEs
- Enhance maintenance practices
- Digital transformation: Customer insight matters
- IoT digital experiments as a service
- Smarter maintenance: Paper available
- Condition monitoring & Industry 4.0
- Smart data collection
- Mechanised welding, cutting and Industry 4.0
- Tomorrow’s tech is here today
- The business value of Industry 4.0